A DOE User Facility, Features A Unique Particle Accelerator That Nuclear Physicists Use To Explore The Heart Of Matter
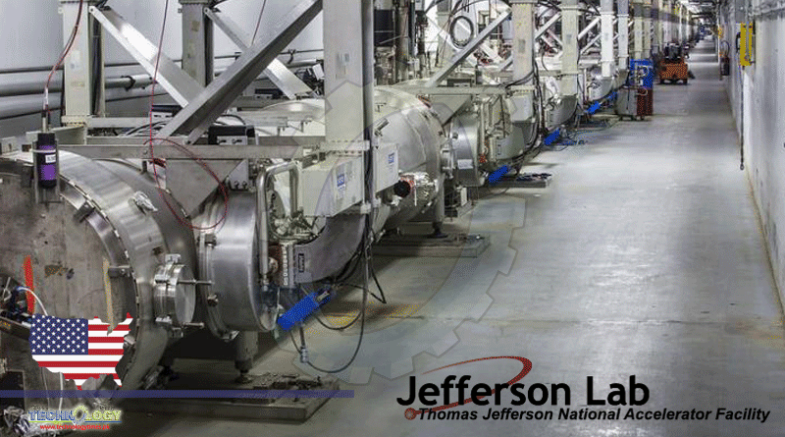
Operators of the primary particle accelerator at the U.S. Department of Energy’s Thomas Jefferson National Accelerator Facility are getting a new tool to help them quickly address issues that can prevent it from running smoothly. A new machine learning system has passed its first two-week test, correctly identifying glitchy accelerator components and the type of glitches they’re experiencing in near-real-time. An analysis of the results of the first field test of the custom-built machine learning system was recently published in Physical Review Accelerators and Beams.
The Continuous Electron Beam Accelerator Facility, a DOE User Facility, features a unique particle accelerator that nuclear physicists use to explore the heart of matter. CEBAF is powered by superconducting radiofrequency cavities, which are structures that enable CEBAF to impart energy to beams of electrons for experiments. “The heart of the machine is these SRF cavities, and quite often, these will trip. When they trip, we’d like to know how to respond to those trips. The trick is understanding more about the trip: which cavity has tripped and what kind of fault it was,” said Chris Tennant, a Jefferson Lab staff scientist in the Center for Advanced Studies of Accelerators. Expert accelerator scientists review information on these faults and can use that to determine where the fault started and what type of fault it is, thus informing CEBAF operators on the best way to recover from the fault and mitigate future ones. However, that expert review takes time that operators don’t have when experiments are underway.
In late 2019, Tennant and a team of CEBAF accelerator experts set out to build a machine learning system to perform that review in real-time. They worked with several different groups to design and build from scratch a custom data acquisition system to pull information on cavity performance from a digital low-level RF system that is installed on the newest sections of particle accelerator in CEBAF, which includes about one-fifth of the SRF cavities in CEBAF. The low-level RF system constantly measures the field in SRF cavities and tweaks the signal for each one to ensure that they operate optimally. When a cavity faults, the machine learning data acquisition system pulls 17 different signals for each cavity from the digital low-level RF system for analysis. “We’re leveraging information-rich data and turning it into actionable information,” he said. These same information-rich data are used by accelerator experts to help identify faulting cavities and causes. These past analyses were used to train the machine learning system prior to deployment.
The new system was installed and tested during CEBAF operations over an initial two-week period in early March 2020. “For that two weeks, we had a few hundred faults that we were able to analyze, and we found that our machine learning models were accurate to 85% for which cavity faulted first and 78% in identifying the type of fault, so this is about as well as a single subject matter expert,” Tennant explained. This near-real-time feedback means that CEBAF operators can take immediate steps to mitigate problems that arise in the machine during experimental runs, and hopefully preventing smaller problems from turning into bigger ones that can reduce experiments’ runtime. “The idea is eventually, the subject matter experts won’t need to spend all their time looking at the data themselves to identify faults,” he said.
The next step for Tennant and his team is to analyze data from a second and longer test period that took place in late summer. If the system performed as well as the first test indicates, the team hopes to begin designs for extending the system to include older SRF cavities in CEBAF. This project was originally proposed and funded through Jefferson Lab’s Laboratory Directed Research & Development program for fiscal year 2020, and it was later selected by DOE for a $1.35 million grant to leverage machine learning to revolutionize experimentation and operations at user facilities in the coming years. “This was a proof-of-principle project. It was somewhat riskier, because several years ago, when this project was proposed, none of us on the team knew anything about machine learning. We just sort of jumped in,” Tennant said. “So, sometimes supporting those higher-risk/higher-reward projects really pays off.”
This news was originally published at Eurek Alert