Scientists have developed a new anti-counterfeiting technology that they say can better ensure the authenticity of high-value products.
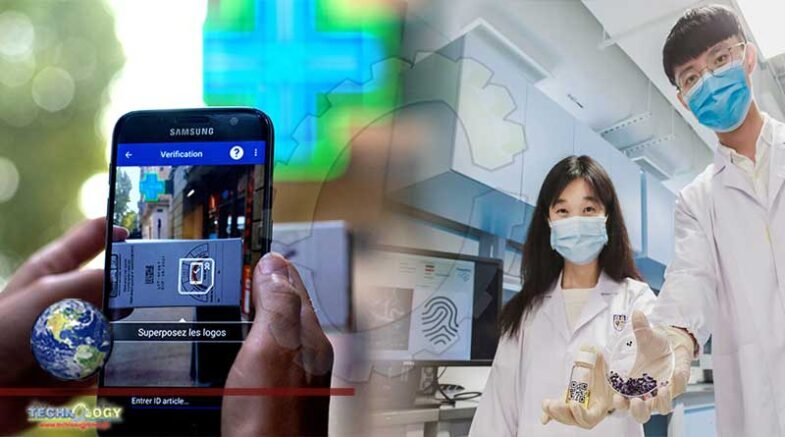
Scientists at the National University of Singapore (NUS) have developed a new anti-counterfeiting technology they say can better ensure the authenticity of high-value products such as jewelry, electronics and pharmaceuticals. Called DeepKey, the technique relies on a mix of crumpled two-dimensional films and deep learning to offer a high level of durability and very fast authentication.
DeepKey is based on Physically Unclonable Function (PUF) patterns, which are unique physical identifiers impossible to copy that are currently used in some existing anti-counterfeiting efforts, such as in microprocessors. However, the NUS researchers say manufacturing PUF tags can be complicated and they can suffer from environmental instability and long authentication times, and they believe they have found a better way forward, beginning with a simple balloon.
“First, we inflate the balloon and brush over its surface with viscous 2D-material ink,” explains Dr Jing Lin, a member of the research team. “After air-drying overnight, we deflate the balloon. Because of the interfacial mechanical mismatch between the 2D-material and latex substrate, large-area, crumpled PUF patterns are generated during the contraction. These PUF patterns can be cut to the required size afterwards, and normally, hundreds of them can be made at one time.
These patterns are then imaged with an electron microscope, which deep learning software then analyzes to classify and validate the unique pattern in minutes. This marks a significant step forward in the eyes of the team, compared to today’s PUF-based technologies where the patterns need to be authenticated by searching through a single, huge database, which limits their use.
“The whole process takes less than 3.5 minutes, most of which is spent waiting for the readout from the electron microscope,” says Jing. “The authentication itself is very fast, in less than 20 seconds.”
This comes down to distinct features on the patterns that the deep learning software, after being trained, is able to more easily recognize.
“We used the deep learning model to pre-categorize the PUF patterns into subgroups, and so the search-and-compare algorithm is conducted in a much smaller database, which shortens the overall authentication time,” explains Jing.
In addition to this faster authentication time, the team says its DeepKey tech is more durable and not so easily damaged by things like extreme temperatures, UV exposure and moisture. They note its potential for authenticating vaccines, some of which need to be stored at very cold temperatures.
“With this research, we have tackled several bottlenecks that other techniques encounter,” says study author Wang Xiaonan. “Our 2D-material PUF tags are environmentally stable, easy to read, simple and inexpensive to make. In particular, the adoption of deep learning accelerated the overall authentication significantly, pushing our invention one step further to practical application.”
The team is now continuing its work in hope of shortening the authentication times even further.
Originally published at News Atlas