Solar power and advanced computing are a key cleantech intersection point. From renewables return on investment optimization to optimal rooftop commercial solar deployment, machine learning is helping us get more efficient and effective in our global transformation.
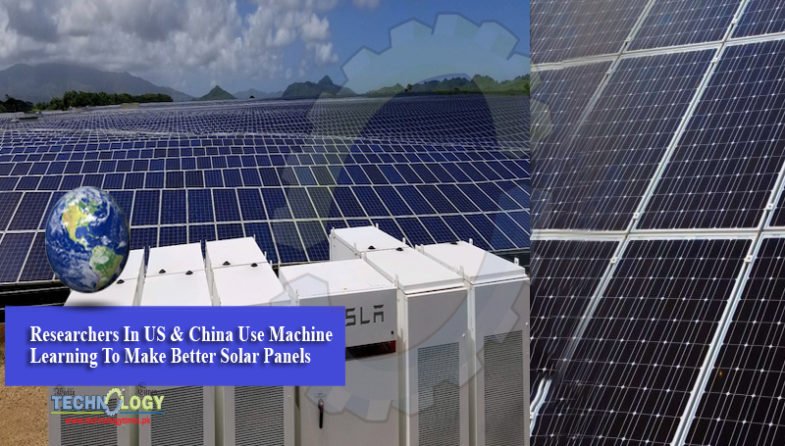
But it’s deeper than that. Researchers in the US and China are using machine learning to discover new solar panel chemistries to increase the base efficiency and economic effectiveness of solar panels. They are trialing hundreds or thousands of combinations in virtual test beds before bringing them into the physical world, a key element of the machine-to-reality value proposition.
Let’s start in the United States with Jinxin Li, Basudev Pradhan, Surya Gaur, and Jayan Thomas from the sun-drenched campus of the University of Central Florida. Their focus is on perovskite solar panels. This set of chemistries featuring inorganic and organic elements has achieved efficiencies of 28%, exceeding standard silicon maximum efficiencies, and is advancing quickly. However, the options for composition are very large, and the number of researchers limited. In their study, published under the title Predictions and Strategies Learned from Machine Learning to Develop High‐Performing Perovskite Solar Cells in Wiley, the team attempted to simplify this process. They used 333 data points from about 2,000 peer-reviewed studies on the subject to train a model to look for higher probability chemistries. They’ve not only identified the most likely candidates for physical research, but with reasonable accuracy predicted performance based on underlying chemical factors. Their work supports and suggests approaching the theoretical Shockley-Queisser limit for individual solar cell efficiency as well. While solar panels have diminished in price mostly due to massive economies of scale for manufacturing, distribution and construction, increased efficiency with cheap and plentiful minerals still has room to bear fruit.
Also in the US are Baskar Ganapathysubramanian and team at Iowa State University, supported by grants from the Department of Energy’s Advanced Research Projects Agency – Energy (ARPA-E). The extended team spans the United States with New York University, California’s Stanford University, and a member from NREL in Colorado. Their focus is on the application of machine learning to organic, thin-film solar panels. This subset of solar technology has been less efficient than conventional solar panels and the perovskite chemistries, but the benefit is highlighted in the name. Thinness equates to lightness, flexibility, and even transparency. They’ve been used in integrated photovoltaics in consumer devices, window-pane layers which generate electricity and conventional solar farms, but they’ve been in decline in recent years in favor of more efficient technologies. They too have room to improve, but run into the same problem of the vast number of compositional choices that can be made, something difficult to deal with through conventional research approaches. Enter Ganapathysubramanian’s team. Their intent is the same as the perovskite-focused researchers, to find chemistries which are higher efficiency and cheaper to manufacture. It’s early days for them, and their work is in aid of a larger goal of applying the approach to multiple domains.
The next team working on solar technology with machine learning approaches are Wenbo Sun, Yujie Zheng, et al., out of Chongqing University, the North China University of Science and Technology, and the Chinese Academy of Sciences. Their focus is on the emerging space of organic solar cells, which use organic polymers or molecules to capture the energy of the sun and convert it to useful electricity. Efficiency is lower still in this space so far, with maximum efficiencies under 20% to date. But the range of organic chemistries is vast, it’s a new field, and the upsides are large if they can be made more efficient and durable. Once again, this is a light, thin-film technology and inherently flexible and fast to manufacture. And once again, machine learning comes to the rescue. As the approach requires data and organic films are relatively new, the team first started by assembling a massive dataset of over 1,700 materials from literature. They then explored how to fingerprint the molecular structures most effectively for application of machine learning approaches, and finally verified it against a new set of materials to assess predictive quality. And predictive quality is good. Expect more advances from this team in this solar technology over the coming years, and a new breed of thin, light, and cheap solar panels to emerge from China because of it.
The world is vast, and the chemicals and organic compounds within it innumerable and dizzying in their combinations and permutations. Machine learning is helping researchers find the highest probability combinations for more efficient and cheaper solar technology, something which will benefit everyone in the world.